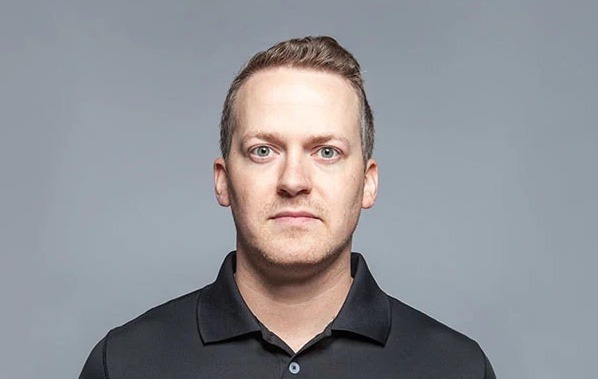
30
Luke Bornn
The future of data science in football
August 23, 2021
LUKE BORNN is one of the pioneers of data science in sport.
After working as a Professor of Statistics at Harvard University, the Canadian became Head of Analytics at AS Roma in Italy, where he played a role in the key signings of Alisson Becker, Antonio Rüdiger and Mo Salah.
A stint in the NBA, as Vice President of Strategy and Analytics for the Sacramento Kings, followed, and Bornn is now the founder and chief scientist for Zelus Analytics, which is making some big signings of its own.
Bornn was our guest on Episode #30 of the TGG Podcast and told us about his own journey, and that of analytics in football. You can listen, and read an edited transcript, below.
1. Keeping the message simple
Luke Bornn: Probably the biggest mistake most analysts make when they go into a club is they overwhelm everyone around them with way too much information. At Toulouse now, I push our guys really hard to say, ‘Look, our posting report, I would be totally happy if it was a blank page with a single number in the middle of it.’
For the most part, they want to do more and more and it’s just overwhelming. A good reference point is to think about my time at the Sacramento Kings. I spent a little over three years there. I have a PhD in stats and machine learning, my whole background is in building really complex models and understanding really complex processes, and if I think about what I did in my three years at the Kings, ultimately, it was convincing people to take more three-point shots.
Fundamentally, when you boil it down, I was trying to convince people that the number three is bigger than the number two. You don’t need complex maths for any of that stuff. Often, the biggest sources of impact you can have within an organisation are really really simple things.
I always think, ‘What are the levers an organisation can pull to get better?’ Usually, there aren’t that many and if there are, you’ve got to narrow it down to say, ‘Hey, what are the two or three things we need to focus on?’
And you should be able to represent that lever with one or two numbers. There really isn’t any reason to go beyond that.
2. Data for recruitment: Fine-scale estimations
I tend to believe, across sports, that the number one lever teams have to create wins is in recruitment – putting the best players possible on the pitch.
My experience is that scouts are decently good at saying ‘this player is good or bad’, but when there’s a lot of grey area, human valuation is not necessarily good at small levels of differentiation.
When you’re talking about professional sport, small levels of differentiation – a player that adds two wins versus a player that adds four wins – that’s the difference between a player you’re going to pay £10m a year for versus one you’re going to pay £20m for. That’s a huge range.
Paying a player £20m who should only be making £10m – if you do that a couple of times as an organisation, you’re going to be in really big trouble. Statistical methods are really good at those sort of fine-scale estimations, at saying this player is worth between £8m and £12m a year, but definitely not worth £20m a year.
That’s where we’re able to add a lot of value, to say, ‘Ok, let’s understand this player’s skill’ and in really simple terms make sure we’re aligned with scouts and provide context for them, but frame the overall contribution with a lot more precision than us, as humans, are comfortable or confident of doing.
The state of the art in recruitment in football, at least as I experienced it in various clubs, [is] you get a call from an agent saying, ‘My player is available,’ you pull him up on Wyscout, watch him for 20 to 30 minutes, and then make a decision. Literally, I’ve seen that over and over from clubs and it still happens to this day.
It’s only now where organisations are building up the capacity to be able to say, ‘Hey, we can use all this data and insights alongside scout’s information, their eye test on the player.’ But I think most organisations aren’t in a position to be able to handle that.
3. Having the leadership and processes to execute
The thing that caught me off guard the most was how interested teams were in data, but how little the data actually influenced decision making. This has been a pattern of my entire career, observing where you will have an ownership group or board of directors who says, ‘yes we need to invest in data’ – and they might bring in an analyst, pay for some data – and then in the end the impact of this role is almost nil.
That was probably the thing that surprised me the most, how an organisation could say ‘we think this is really important’ but not put in place the leadership or processes to actually execute on that data.
I used to work for Electronic Arts on the Fifa franchise and one of the core areas where I worked was Ultimate Team. One of my roles was to understand various aspects of player behaviour. This was well over 10 years ago.
If I looked up the chain of command, first off there weren’t that many layers above me, and all those people were technical people, very data-driven, and used to thinking about things from an objective perspective.
In most sports teams, if you look above, it’s typically people who grew up playing the sport. It’s nothing malicious, these are just people with very different backgrounds, they are not trained or familiar with how best to collate and leverage different sources of information to make coherent decisions.
It’s fundamentally a difference of background in terms of the people who are typically leading clubs now versus what I experienced when I was working in tech.
In basketball, for the most part, GMs continue to be former players, agents, and analytics and data is still a relatively small part of the overall decision on most players. When I was at the Kings, the senior leadership was two former star players – Vlade Divac and Peja Stojakovic. They were great guys and knew the game inside and out, but just didn’t have experience integrating data into decision making, so that was a lot of my role, thinking how do we best use data to augment and complement decision making.
One of the challenges clubs find is to say, ‘Hey, we want to use data and improve our decision making,’ but it’s a huge world out there – analysts with drastically different skill sets, different data providers, event data, tracking data – so how do you actually decide what are the things that are going to bring the club more wins?
This is, for the most part, where clubs fail. They bring these people in who have no idea of what to listen to or not, and how to direct that focus and attention. In the end it ends up getting ignored completely.
4. A unit of objective throughout a club
One of the biggest things clubs can do is come to a unit of objective. Ultimately, it’s got to be wins and goal differential. Then you can start to frame every question – do we hire a nutritionist or another physio – and you can break it down.
Give me a back-of-the-envelope calculation on what will be the impact on goal differential and wins?
If you look around at most clubs, they are working on really good things, where they’re trying to optimise towards different things, and the problem is, when you think about the executive leadership, it’s really hard to know how to think about prioritising different projects and where to invest your money and time and attention.
A lot of it is about using the experience we have in other sports and businesses to ultimately make better decisions, and that can be on the business and football side, understanding where to invest and not to invest, and what things you can spend your time and attention and money on that will increase the teams chances of winning.
5. Extracting the full nuance of tracking data
If you think about what’s going on on a football pitch, you have 22 actors interacting. You have individual action, interaction between units that are collaborative, adversary actions, interaction of the officials, interplay with the ball, and you get good data on this, where you’re measuring it multiple times per second. You get the locations of the players, the 3D location of the ball and it’s incredibly rich data.
Over the last five or 10 years that data has spawned literally hundreds of papers and pushed forward our understanding of these types of tracking data sets and ultimately led to advances in other domains, like wildlife tracking and defence and military.
Once you go to tracking data you capture all that off-ball information, but also the level of technical sophistication required to extract that kind of info, there is literally not a football club in the world that has the ability to do that to its full extent, not even the biggest analytics groups in football, if you look at Liverpool or Arsenal. To actually fully dive into the raw tracking data and extract the full nuance of all these off-ball actions, no club is there.
6. Creating space (even by standing still)
Football is ultimately a game of space and space ownership, this is one of the things that really differentiates it (from other sports).
We can use this tracking data to understand a player’s ownership of space. One of the easiest ways to measure space is to say, ‘If I dropped a football on a very particular location, which player would be the first to get there?’
For every location on the pitch, which would be the first player to get there? Those types of techniques have become a staple of sports analytics.
I wrote a paper a couple of years ago with Javier Fernandez. We looked at Barcelona and showed that Lionel Messi has this uncanny ability to know when NOT to follow the play. We did this really nice study using the player tracking data, showing he creates a ton of value and space and opportunities for his team-mates when he chooses not to move.
We always think about using movement to create space, in this case it was really interesting because it allowed us to think, ‘Hey, if everyone else is moving, relative to the rest of your team or the opposition, you are moving even if you are standing still.’ This is something clubs and coaches could think more about, runs and also anti-runs.
7. What football can learn from US sports
I think football teams can learn a lot from both basketball and American Football in the design of set pieces. The way that basketball players think about screens, which is essentially getting in the way of your opposition and using your body tactically in a ‘legal’ way. In football this happens all the time, but set pieces aren’t designed in a really deliberate way.
Looking at American Football, can we learn something from how these guys are running routes, because ultimately it’s about creating space and opportunity. If you think about a corner, it’s ultimately about creating moments of space and opportunity for a guy to get a head on the ball.
This is the identical thing American Football coaches have been doing for the last 100 years, which is design co-ordinated actions that open up spaces for receivers to receive the ball.
Also, from a management perspective, really just professionalising the management of these sports teams. Baseball is a great example I always look to, because they’re so far ahead. If you look at the GM (General Manager) hirings in MLB over the last 10 years, they were all former McKinsey consultants and Harvard MBAs, it’s just such a different world now than it was.
In the end, you end up with front offices which much more closely resemble businesses.
8. Machine learning
Machine learning is a core piece of what we do. Maybe the best way to think about machine learning is using data to try and predict or understand some outcome. We can talk about it being the widget that takes all the tracking data and predicts the probability of scoring.
What we do is say, ‘We have all this tracking data, we are going to decompose it in a way where when it comes out it doesn’t just say Messi is good, it says he is good in these 30 characteristics, maybe ok in these four and bad in these two.’
The key thing there is that allows you to say this player is playing in the wrong context. Teams have people on staff that have those (machine learning) skills, but for the most part, if you look at what they are actively using to impact decision making, it’s relatively simple things, like looking at a striker and asking how many expected goals he had and going further and looking at expected assists, so simple count metrics.
There are one or two in the world but not many that are using machine learning, which is not surprising when you think about the level of complicity it takes, the level of technical skill it requires to build those types of models and then, ultimately, the challenge with interpreting and integrating into a decision-making process.
9. A new way for sports analytics?
In the NBA, the average analytics team is about four or five people, in baseball it’s probably about eight to 10. The whole premise of Zelus is that even with 10 people on staff, teams don’t have the bandwidth to deal with the raw tracking data and do the full-fledged player valuation you would want to do with this kind of data.
Because there are always demands on their time, from pre-game reporting, ad hoc requests etc, even the really big groups in baseball don’t necessarily have the right combinations of staff to deal with this tracking data and do it in the right way.
When we think about building these types of models in basketball, our estimation of the amount of work is 30 person years. What football club can devote 30 person years to a statistical modelling task? The answer is obviously none.
My focus tends to be aligned in two ways. One is on the technical side – how do we get the best valuation on athletes and on winning? How do we fuse data with machine learning and statistical models that turn it into actionable intelligence and value and how do we combine that with other sources of information like intel and contract information to get the best possible valuation for players?
The second thing is how do we build an organisation that makes consistently high-quality, good process-driven decisions and decisions that are ultimately going to lead to success? You’re always flipping the coin and trying to bias that coin a little bit to make slightly better decisions every time. If you do that repeatedly, those slightly better decisions add up into long-term sustainable advantage.
Zelus have grown relatively quickly. We are an insights company working across sports and RedBird is our primary financial partner. My main focus is with Zelus and Toulouse.
We took over Toulouse a little over a year ago and are really happy with where we are. Damien Comolli and Olivier Sadran have been guiding the ship and we’ve been really happy with where that’s at.
We are not out there selling to every team, we have a very small exclusive set of partners who are partnering with us long term. They essentially hold exclusivity with us in perpetuity. That means we can think of ourselves in some sense as an extension of their internal analytics group.
Because of this long-term partnership, we want our teams to be able to understand and leverage our information as much as possible.